Can AI Revolutionise Field Engineering and the Service Sector?
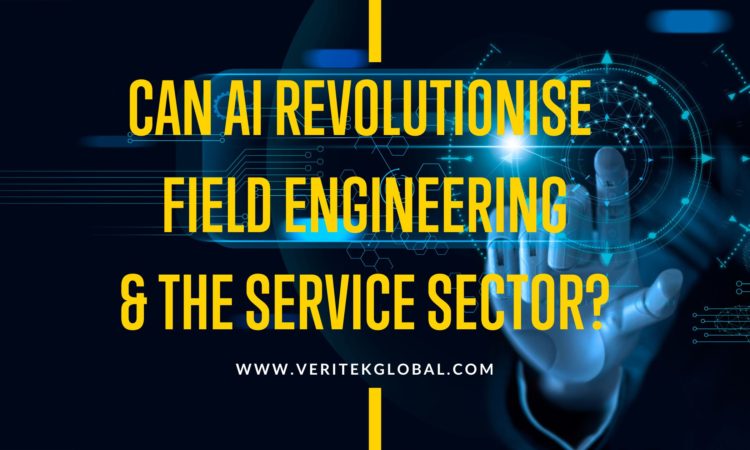
Integrating AI into after-sales service operations represents a thought-provoking development in the sector’s approach to problem-solving and efficiency. In an interview with Veritek’s Sales and Marketing Director, Adrian Clark, we explored the potential of AI in after-sales services, particularly within original equipment manufacturers (OEMs) and independent service organisations like Veritek.
Field engineering has long relied on institutional memory and knowledge bases to support group-wide learning and to understand the root causes of problems and trends where they occur.
Veritek, for instance, maintains a robust knowledge base of encountered faults, facilitating learning and problem resolution across the organisation.
In practice, this means that if an engineer sees a particular type of output failure from a device, he will know ten engineers encountered the same problem. Moreover, the problem related to ‘widget X’ in seven cases. In that case, it makes sense to investigate ‘widget X first.’
By leveraging AI, engineers can improve the speed and quality of diagnosis and resolution by analysing data to identify trends, predict issues before they occur, and optimise processes.
How does AI work?
AI draws upon tremendous processing power and information. It can see trends, connections, and causalities that humans cannot see and quickly process.
This iterative learning process enables rapid identification of recurring faults and optimal troubleshooting strategies, leading to more efficient service delivery.
AI’s capabilities extend beyond traditional problem-solving. Its ability to recognise patterns, solve complex problems, and learn from experience means it can also predict failures based on usage, lifespan, environment, or other factors, enabling predictive maintenance and trend analysis.
Beyond that, AI can streamline diagnostics and optimise stock management by analysing vast datasets encompassing service events, equipment specifications, and environmental factors.
This proactive approach enhances equipment uptime and reduces operational costs for OEMs and end-users.
For instance, AI-driven diagnostics can pre-emptively identify issues, enabling timely repairs and mitigating the risk of unexpected breakdowns.
Furthermore, by optimising scheduling and resource allocation, AI enhances service efficiency and response times, enhancing customer experience and satisfaction.
How AI could work in practice in a service organisation
Let’s look at a print and graphics OEM, for example.
The longer its product is live, the more consumables are consumed and the more money the customer and OEM make.
If the OEM can predict failures and pre-emptively fix problems, it will improve customer experience.
Providing a good customer experience translates into more loyalty and more chance of repeat business.
Then, if the OEM can plan maintenance and break/fix visits to pre-empt failures, which is more cost-effective than annual maintenance, everyone will save money.
If an OEM can improve the probability that an engineer fixes issues the first time because AI has told the field engineer which three parts to take that are 99% likely to be the parts needed. You will only have one engineer visit, not multiple visits.
After that, if you look at an OEM’s cost to deliver service, better analysis and understanding of issues can help it to fix more problems remotely.
Not having an engineer visit customers’ sites reduces the environmental impact of travel.
Ultimately, AI can reduce costs related to servicing events and improve customer experience.
Finally, a better and broader data analysis about failures aid service organisations in improving all those incremental things we’re constantly seeking to enhance from a service standpoint.
Challenges in the adoption of AI in after-sales services
While AI holds immense potential, its adoption poses challenges, particularly regarding data quality and volume.
Effective AI implementation necessitates comprehensive historical data and ongoing refinement to ensure accurate decision-making.
Additionally, the economic viability of AI initiatives varies based on equipment volume and complexity, requiring careful consideration of return on investment.
To illustrate, if you want AI to tell you about smartphone failures, you can extract data from millions of connected smartphones if the user allows it.
But if you want AI to improve the quality of a Hadron collider. In that case, it’s probably not worthwhile because only a few exist worldwide and are all different. So, AI cannot draw upon a vast database of information.
Despite these challenges, AI has the potential to offer unprecedented insights, operational efficiencies, and cost savings in the after-sales service sector.
As OEMs embrace AI-driven technologies in product development to enhance functionality, performance, and user experience, they gain from operational efficiency, data-driven insights, customer satisfaction, loyalty, and repeat business.
Moreover, as AI evolves, it will necessitate ongoing adaptation and integration into existing workflows and business models.
In the meantime, OEMs must address emerging concerns such as data privacy, algorithmic bias, and the need for human oversight in critical decision-making processes. Especially in complex and unpredictable service situations where human judgment and empathy are valued.
In essence, AI represents a powerful ally in pursuing service excellence and operational efficiency.
By harnessing its capabilities effectively, in the future, OEMs and service providers can unlock new opportunities for growth, customer value creation and competitive advantage by way of fast, personalised service and increased efficiency.